Deep-learning-based image analysis is now just a click away
10/14/21
Researchers from the Universidad Carlos III de Madrid (UC3M), the Instituto de Investigación Sanitaria Gregorio Marañón (IiSGM), and collaborators in Switzerland and Sweden, have developed a tool, called deepImageJ. The tools processes and analyses using models based on artificial intelligence biomedical images (for example, acquired with microscopes or radiological scanners), improving their quality or identifying and classifying specific elements in them, among other tasks.
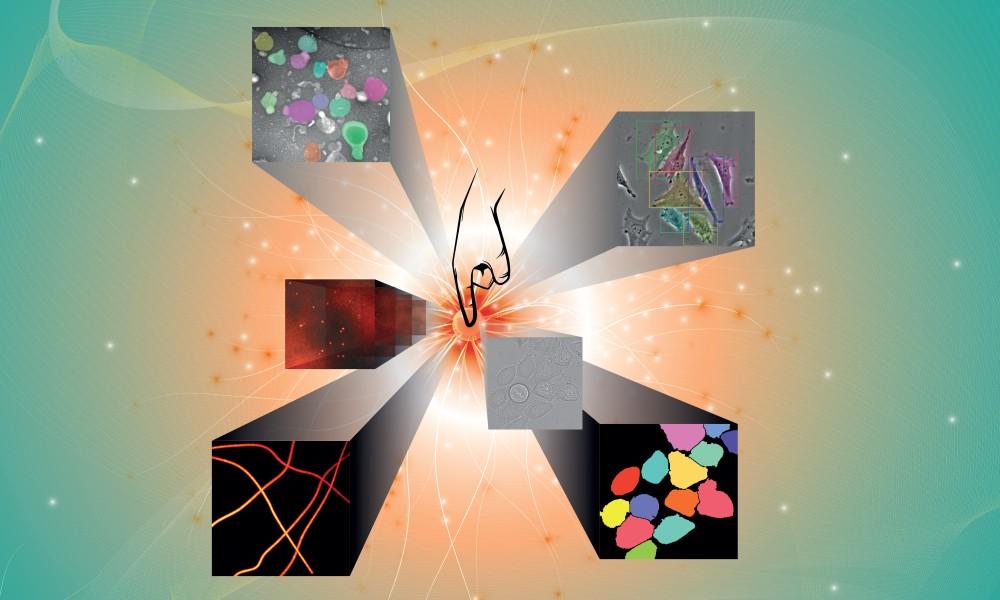
Deep-learning models are a significant breakthrough for the many fields that rely on imaging, such as diagnostics and drug development. In bio-imaging, for example, deep learning can be used to process vast collections of images and detect lesions in organic tissue, identify synapses between nerve cells, and determine the structure of cell membranes and nuclei.
“Over the past five years, image analysis has been shifting away from traditional mathematical- and observational-based methods towards data-driven processing and artificial intelligence. This major development makes detecting and identifying valuable information in images easier, faster, and increasingly automated in almost every research field. When it comes to life science, deep-learning-, a subfield of artificial intelligence, is showing an increasing potential for bioimage analysis. Unfortunately, using the deep-learning models often requires coding skills that few life scientists possess. To make the process easier, image analysis experts from several institutions have developed deepImageJ. An open-source plugin described in a paper published this month in Nature Methods", explains one of the project's principal investigators, Arrate Muñoz Barrutia. She is a professor at UC3M Department of Bioengineering and Aerospace Engineering and senior researcher at IISGM.
Using neural networks in biomedical research
This type of artificial intelligence involves training a computer to perform a task by drawing on large amounts of previously annotated data. It is like CCTV systems that perform facial recognition or mobile-camera apps that enhance photos. Sophisticated computational architectures called artificial neural networks are the basis of deep-learning models. Multiple processing layers form these networks, and the layers can mathematically model the data at different levels of abstraction.As previously commented, developers train the neural networks to solve specific research purposes, such as recognising certain types of cells or tissue lesions or improving image quality.
Once trained, the information needed to perform the task, called the neural network model, is stored as a structured file in the computer and can be easily reused with deepImageJ. Namely, deepImageJ enables researchers worldwide to apply them with just a few clicks. “This application bridges the gap between artificial neural networks and the researchers who use them. A life-sciences researcher can now ask an IT engineer to design and train an automatic learning algorithm to carry out a specific task. The scientist can then use the development easily through a user interface, without seeing a single line of code,”observedDaniel Sage. He’s a researcher from the École Polytechnique Fédérale de Lausanne(EPFL Center for Imaging) in Switzerland, who is supervising the project’s development.
Open-source, collaborative software
The plugin is released as open-source software and free of charge. It is a collaborative resource that enables engineers, computer scientists, mathematicians and biologists to work together more efficiently. Namely, researchers worldwide can contribute to improving deepImageJ by sharing their user experiences, proposing improvements, and requiring updates.
“Our objective is for this resource to be used more and more by researchers from any conventional computer and without needing to have any programming knowledge. So that as many researchers can use the plugin as possible, our research team is also developing virtual seminars, training material, and online resources. The materials are designed with both programmers and life scientists in mind so that users can quickly come to grips with the new method. The more users who employ the tool, the more interaction between developers and biomedical researchers will be enhanced. This interaction will thus accelerate the dissemination of new technological developments. Above all, the advancement of biomedical research,” Professor Muñoz Barrutia pointed out.
Publication in Nature Methods
The study’s principal authors are Arrate Muñoz Barrutia (UC3M and IISGM) and Daniel Sage (EPFL Center for Imaging). Estibaliz Gómez de Mariscal (UC3M and IISGM), Carlos García López de Haro (UC3M and IISGM), Michael Unser (EPFL Center for Imaging) and LaurèneDonati (EPFL Center for Imaging), and collaborators from the KTH Royal Institute of Technology inStockholm (Sweden), Wei Ouyang and Emma Lundberg form the collaborators' team. They have been made possible for the deepImageJ project's development and most relevant advances to be published inNature Methods. The latter isa monthly scientific publication reviewed by editors from the Nature Publishing Group,providing relevant information on new scientific techniques and laboratory methods. This work has received financial support fromMinisterio de Ciencia, Innovación y Universidades, AgenciaEstatal de Investigación, of the Spanish Government, European Regional Development fund, the COST action NEUBIAS, 2017 Leonardo grant of the BBVA Foundation, EPFL Center for Imaging, Erling-Persson Family Foundation and Kunt and Alice Wallenger Foundation.
For more information: